There’s a well-documented model known as the DIKW Pyramid, that depicts superficially the hierarchy/relationship structure between Data, Information, Knowledge and Wisdom. Its origins date back to the 1950s, though the hierarchy itself was formalised in the early 1980s.
But what is it actually telling us? And, more importantly, how are organisations applying the insight it gives us about these relationships to improve how they operate?
Let’s start with some basic definitions:
Data: a set of “discrete, objective facts or observations, which are unorganised und unprocessed and therefore have no meaning or value because of a lack of context and interpretation” (1)
Information: “organised or structured data, which has been processed in such a way that the information now has relevance for a specific purpose or context, and is therefore meaningful, valuable, useful and relevant” (1)
Knowledge: a fluid mix of framed experience, values, contextual information, expert insight and grounded intuition that provides an environment and framework for evaluating and incorporating new experiences and information. In organisations it often becomes embedded not only in documents and repositories but also in organizational routines, processes, practices and norms. (2)
Wisdom: the ability to increase effectiveness. Wisdom adds value, which requires the mental function that we call judgment. The ethical and aesthetic values that this implies are inherent to the actor and are unique and personal. (1)
Mmmm…
So, given that in most organisations these terms are used either completely differently or reasonably interchangeably, we have something of a nomenclature issue before we’ve even started working out how to collect, collate, interrogate and enhance mere data, to turn it into much more valuable knowledge and wisdom.
Levels of Sophistication
1. Data Management
First off, we need to understand what data we have within our organisations, where it comes from, where it goes to and how it’s used. This is no mean feat and is often overlooked when organisations embark on what they optimistically call a “data strategy”. Once this has been mapped, a governance infrastructure can be created around it. This oftentimes requires that we re-think how the data flows within our organisation need to work to better serve those that interact with it. There’s also the question or data quality in this part of the jigsaw: remember the old adage? Rubbish in, rubbish out…
By way of anecdote, I’m going to use a supply chain example. In this instance, having data on each and every SKU in a warehouse (what the product is and other specification data, who supplied it, when it was delivered, what its use by date is, how many units are in a pack, etc.) would give me that base level of data – useful stuff to know, and better that I actually have access to it, but there’s no context around what I’m actually going to do with that data now I’ve got it.
2. Data Exploration – Data to Information
This is the “what is the data telling us about?” bit, or better put, identifying the levers, clusters and networks that are pertinent to it. Once we have data flowing correctly around the organisation, so it can be tapped and called on by those that need to query it, we need to be able to support those queries by having the relevant query tools. We then need to empower and enable folks across the organisation to be able to query the data themselves, to self-service their insight needs and reduce their dependency on specialist insight teams and data scientists. That’s easier said than done, as anyone who’s ever done any data exploration will tell you. Most of the time spent on this phase is actually in the data preparation and staging bit. And the only way to really reduce the emphasis on this stage, is to ensure that the data management piece of the jigsaw is a finely honed and tuned process.
Back to the supply chain example. Aggregating all that previous stock data, I can now see how many units of a particular SKU I have in stock, where it is in my warehouse, how long it’s been there and how long I have before I have to move or sell it. What’s lacking here though is taking any action because I have this level of information.
3. Data Analytics and Visualisation – Information to Knowledge
The ability to slice and dice the data and start sharing (sometimes referred to as “surfacing”) the insights across the organisation, gives us the bridge between simply having information sitting there, and operationalising it in the form of getting the insights contained within it to the right people in a timely and relevant way so that they can do something about it. This is predominantly descriptive and diagnostic analysis.
Now I’m analysing my supply chain information by surfacing the insights in a series of reports and dashboards. I’m using this to then make a series of informed decisions about re-stocking my warehouse with particular SKUs. I’m also ensuring that other articles that are soon to be past their sell by dates are flagged to marketing. This allows my marketing colleagues to run appropriate promotions to shift that stock so that I don’t end up with perished goods that I have to write off.
4. Predictive Analytics and Process Optimisation – Knowledge to Wisdom
This last level of sophistication allows organisations to really drive competitive advantage. It’s about pre-empting the need for someone or some process to intervene though a series of alerts or alarms to effect a change. Instead, by analysing many data with the use of predictive analytical algorithms and techniques, we are able to forecast what will happen with a reasonable level of confidence.
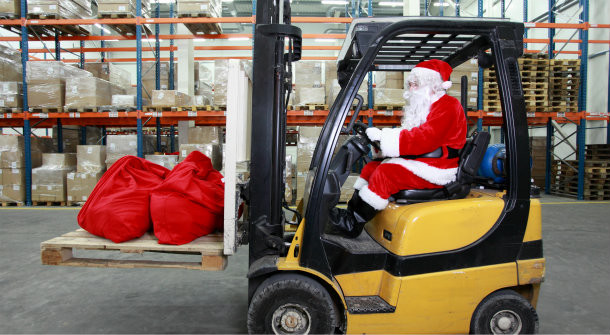
Now, let’s imagine it’s coming up to Chirstmas in our scenario. Having analysed last year’s stock numbers and sales results, the level of interest in particular sets of toys on social networks and the marketing campaigns supporting them, the general level of confidence amongst the public over the economic outlook, and the level of affluence by store catchment area, I can build a robust model and forecast how many toys I need to order in for the whole of the UK. I can also accurately predict what quantities of each one to send to my various stores across the country, as well as how many I will likely need to satisfy internet sales up to the postal cut-off point.
Makes you wonder why Santa has always been portrayed as a wise old chap who knows exactly what every child wants and manages to get it to them just in time for Christmas, doesn’t it?
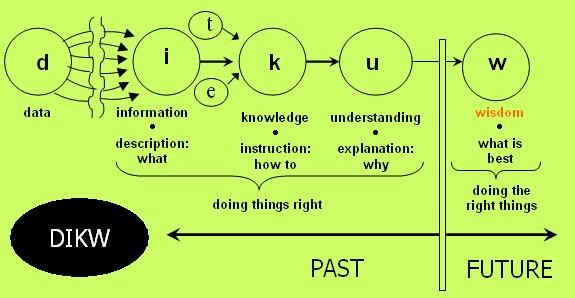
(1) Jennifer Rowley, Organising Knowledge: An Introduction to Managing Access to Information, 2006
(2) Thomas H Davenport, Working Knowledge: How Organisations Manage What They Know, 1998
(3) Picture: http://upload.wikimedia.org/wikipedia/en/thumb/9/93/DIKW.png/300px-DIKW.png